Alcohol: Are happy hours good for your heart?
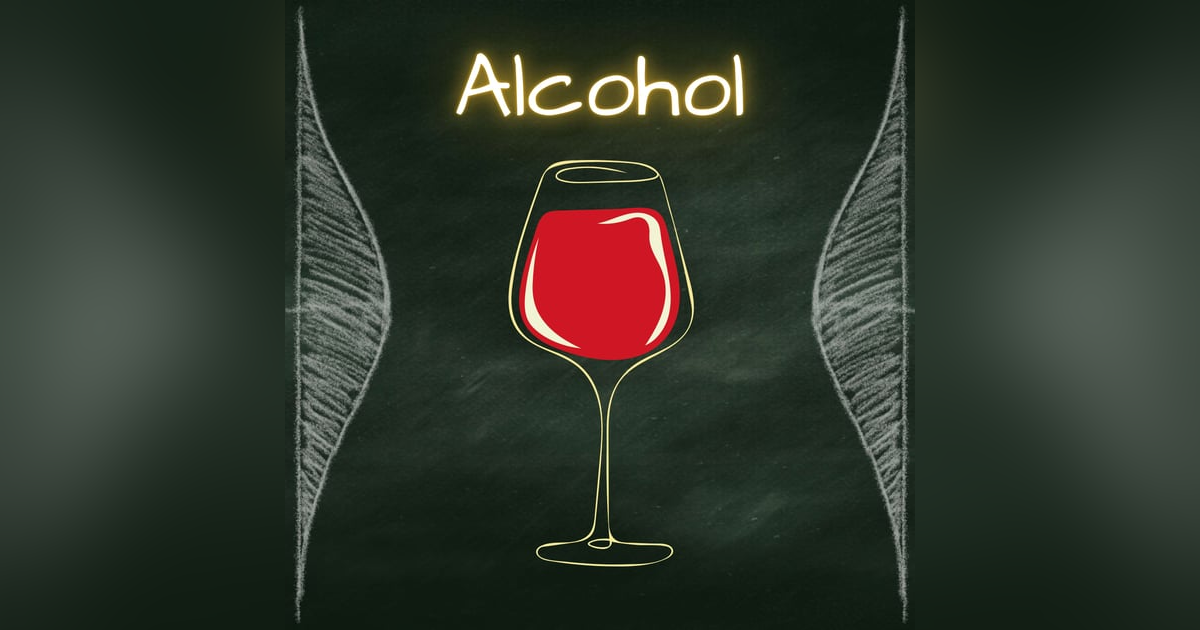
Does a daily glass of wine really keep the cardiologist away? It’s a claim we’ve all heard: light to moderate drinking is good for your heart. But is it science or just a convenient excuse for happy hour? In this episode, we dive into the history behind this claim, discuss the challenges of observational studies and statistical adjustment, and explore attempts at randomized trials and natural experiments to get to the bottom of this boozy debate. Grab your drink—or maybe don’t—and join us!
Statistical topics
- Statistical Adjustment
- Regression
- Residual and Unmeasured Confounding
- Randomized Trials
- Multiple Testing
- Outcome Switching
- Mendelian Randomization
Methodological morals
“Statistical adjustment cannot erase all confounding.”
“When you can’t experiment on people, let Nature experiment on people.”
Citations
- Alcohol and Drinking. Gallup Poll. 2024.
- Canada’s Guidance on Alcohol and Health. Canadian Centre on Substance Use and Addiction. 2023.
- U.S. Department of Agriculture and U.S. Department of Health and Human Services. 2020 – 2025 Dietary Guidelines for Americans. 2020.
- U.S. Department of Agriculture and U.S. Department of Health and Human Services. Alcohol and Cancer Risk: The US Surgeon General's Advisory. 2025.
- Mezue K, Osborne MT, Abohashem S, et al. Reduced stress-related neural network activity mediates the effect of alcohol on cardiovascular risk. JACC. 2023;81:2315-25.
- McPhillips D, Goodman B. Small amounts of alcohol may turn down stress in the brain, benefiting the heart, new study finds. CNN. June 12, 2023.
- Friedman GD, Klatsky AL. Is alcohol good for your health? NEJM. 1993;329:1882-3.
- Sainani K. Alcohol and Weight. Allure. July 14, 2010.
- Wang LU, Lee IM, Manson JE, et al. Alcohol consumption, weight gain, and risk of becoming overweight in middle-aged and older women. Arch Intern Med. 2010;170:453-61.
- Sainani K. Drinking and Weight. Allure. Oct 31, 2008.
- Tolstrup JS, Halkjær J, Heitmann BL, et al. Alcohol drinking frequency in relation to subsequent changes in waist circumference. Am J Clin Nutr. 2008;87:957-63.
- Rabin RC. Major Study on Drinking Will be Shut Down. New York Times. June 15, 2018.
- Mitchell G, Lesch M, McCambridge J. Alcohol industry involvement in the moderate alcohol and cardiovascular health trial. AJPH. 2020;110:485-8.
- Gepner Y, Golan R, Harman-Boehm I, et al. Effects of Initiating Moderate Alcohol Intake on Cardiometabolic Risk in Adults With Type 2 Diabetes: A 2-Year Randomized, Controlled Trial. Ann Intern Med. 2015;163:569-79.
- Slade E, Drysdale H, Goldacre B, et al. Discrepancies between prespecified and reported outcomes. Ann Intern Med. 2016;164:374.
- Biddinger KJ, Emdin CA, Haas ME, et al. Association of habitual alcohol intake with risk of cardiovascular disease. JAMA Netw Open. 2022;5:e223849.
Page with more details on the CASCADE trial
Kristin and Regina’s online courses:
- Demystifying Data: A Modern Approach to Statistical Understanding
- Clinical Trials: Design, Strategy, and Analysis
- Medical Statistics Certificate Program
- Writing in the Sciences
- Epidemiology and Clinical Research Graduate Certificate Program
Program that we teach in:
Epidemiology and Clinical Research Graduate Certificate Program
Find us on:
Kristin - LinkedIn & Twitter/X
Regina - LinkedIn & ReginaNuzzo.com
- (00:00) - Introduction
- (03:00) - Drinking habits in America
- (04:13) - New Canadian drinking guidelines
- (07:51) - Definition of light-to-moderate drinking
- (08:43) - Risks and benefits of light-to-moderate drinking
- (11:37) - History of the heart health claim
- (18:34) - Problems with observational studies
- (22:40) - Statistical adjustment
- (25:39) - Residual and unmeasured confounding
- (31:19) - Overconfidence in observational studies
- (35:16) - Randomized trials of alcohol
- (36:32) - Canceled NIH randomized trial of alcohol
- (41:42) - The CASCADE randomized trial of wine
- (43:18) - The problem of multiple testing
- (47:56) - Outcome switching
- (49:32) - Mendelian randomization
- (59:04) - Mendelian randomization studies of alcohol and heart disease
- (01:03:09) - Wrap-up
00:00 - Introduction
03:00 - Drinking habits in America
04:13 - New Canadian drinking guidelines
07:51 - Definition of light-to-moderate drinking
08:43 - Risks and benefits of light-to-moderate drinking
11:37 - History of the heart health claim
18:34 - Problems with observational studies
22:40 - Statistical adjustment
25:39 - Residual and unmeasured confounding
31:19 - Overconfidence in observational studies
35:16 - Randomized trials of alcohol
36:32 - Canceled NIH randomized trial of alcohol
41:42 - The CASCADE randomized trial of wine
43:18 - The problem of multiple testing
47:56 - Outcome switching
49:32 - Mendelian randomization
59:04 - Mendelian randomization studies of alcohol and heart disease
01:03:09 - Wrap-up
[Regina] (0:00 - 0:04)
Okay. Go ahead.
[Kristin]
Botox is an effective tool. It works.
[Regina] (0:04 - 0:05)
Yeah.
[Kristin] (0:05 - 0:46)
Similarly, statistical adjustment, also an effective tool. It works. But nobody expects Botox to make you completely wrinkle free, and yet everybody expects statistical adjustment to make you confounder free.
Welcome to Normal Curves. This is a podcast for anyone who wants to learn about scientific studies and the statistics behind them. It's like a journal club, except we pick topics that are fun, relevant, and sometimes a little spicy.
We evaluate the evidence, and we also give you the tools that you need to evaluate scientific studies on your own. I'm Kristin Sainani. I'm a professor at Stanford University.
[Regina] (0:46 - 0:52)
And I'm Regina Nuzzo. I'm a professor at Gallaudet University and part-time lecturer at Stanford.
[Kristin] (0:52 - 0:57)
We are not medical doctors. We are PhDs. So nothing in this podcast should be construed as medical advice.
[Regina] (0:57 - 1:03)
Also, this podcast is separate from our day jobs at Stanford and Gallaudet University.
[Kristin] (1:03 - 1:51)
Before we get into the episode today, Regina, I want to thank listeners who have sent us questions. We will be adding a segment to some future episodes in which we address listener questions. You can submit questions at our website, normalcurves.com, under Contact.
Regina, today we're going to talk about drinking. Specifically, we're going to examine the claim that light to moderate drinking is good for the heart. Pretty much everyone agrees heavy drinking is bad for you, but there's been a longstanding belief that drinking small to moderate amounts of alcohol regularly is good for the heart.
Today we're going to do a deep dive into the evidence behind this claim. We'll uncover some history, tackle the limitations of observational studies and what's called statistical adjustment, and examine attempts to do randomized trials or natural experiments to test this claim.
[Regina] (1:51 - 2:05)
Hmm. Kristin, first of all, I love that we are talking about drinking, but I feel like I need to preface this entire thing with something that you already know, and that is I am a lightweight, super lightweight when it comes to drinking.
[Kristin] (2:05 - 2:30)
Yes, Regina, you are a total lightweight. We've actually been drinking together since graduate school. I mean “drinking”, I feel like I should put that in quotes because we were kind of nerdy in grad school.
[Regina]
Kind of nerdy? Very nerdy.
[Kristin]
We were not at bars a lot.
But I was also a lightweight in grad school because I was training for marathons. But even back then, I could still hold my alcohol way better than you.
[Regina] (2:31 - 3:00)
Way better than me. I remember you were so good at it, actually. And I was, you know, the really dorky person off in the corner dumping out my single drink of alcohol in the potted palm, right, in the back of the bar because I couldn't even handle one drink.
You know, I don't know if it's weird or not, but I'm still not a huge drinker, as you know. And depending on who I'm dating or who I'm hanging around with, sometimes I will go weeks or even months without a drink and not miss it. Weird?
What do you think?
[Kristin] (3:00 - 3:59)
Well, actually, it's not weird. Because to prepare for this podcast, I looked up some Gallup poll statistics about drinking habits in Americans.
[Regina]
I was hoping you would say something like that.
[Kristin]
To put it in perspective. I'm going to round really heavily here just to make the numbers simple. But just to give you a sense, about four out of 10 American adults are complete abstainers.
They don't drink at all. That actually surprised me. That's higher than I thought.
[Regina]
Yeah, yeah.
[Kristin]
About two in 10 are like you, so they drink, but infrequently. About three in 10 have one to seven drinks per week.
I used to be in this category. I was previously what you might call a weekend drinker, right, most Fridays and Saturday nights. But I have switched categories. I am now a complete teetotaler. Yep. I'll talk about that more later.
[Regina]
Yep.
[Kristin]
And then about one in 10 is drinking more than seven drinks per week. So you're not weird at all.
[Regina]
Hmm. That's not so bad, actually. A little weird, not too weird.
[Kristin]
You know, you're in good company. Two in 10 like you.
[Regina] (3:59 - 4:13)
Yeah. So I confess, I see these headlines, red wine, good for your heart. And I have often thought, should I start drinking more, right?
Should I learn how to have a nice glass of wine every night to get some of those health benefits?
[Kristin] (4:13 - 4:31)
Regina, I don't think any public health experts would recommend that you take up drinking more. In fact, I don't know if you realize this, but recently, 2023, Canada issued new public health drinking guidelines. And according to Canada, you have the ideal drinking habits.
[Regina] (4:31 - 4:34)
Me? Really? Oh, come on. What do they say? What are the new guidelines?
[Kristin] (4:34 - 4:52)
The new guidelines say that everyone, this is both men and women, should drink no more than two drinks per week.
[Regina]
Whoa.
[Kristin]
And that is a huge drop from their old guidelines, which allowed up to 10 drinks a week for women and up to 15 for men.
[Regina] (4:52 - 4:55)
Hmm. So 10 to 15, down to two? Come on, that is huge.
That is a big drop.
[Kristin] (4:55 - 5:25)
It's a huge jump and obviously a little controversial. It's also a lot less than the current U.S. guidelines. Those guidelines say that women should have no more than one drink per day or about seven per week, and men no more than 14 per week, two a day.
I should mention that the U.S. is revisiting their guidelines, and it's possible they could revise them downwards in the future, but that hasn't happened yet, and I think it'll be very controversial in the U.S.
[Regina] (5:25 - 5:30)
I bet. So what do we mean by a drink? Can we stop and define a drink?
[Kristin] (5:30 - 5:46)
Yeah. It's what scientists call a standard drink. That's five ounces of wine, about a fifth of a bottle of wine, 12 ounces of beer.
That's one can, or 1.5 ounces of hard alcohol like whiskey. That's about a shot.
[Regina]
One shot.
[Kristin]
Yes.
[Regina] (5:46 - 5:55)
So if I go to a restaurant and have a cocktail, or like they give you these big glasses of wine, you're saying that's going to be more than one drink?
[Kristin] (5:55 - 6:16)
That's going to be more than one standard drink.
[Regina]
But it looks like one drink. It's one glass.
[Kristin]
That's right. Well, Regina, you know that I like to shop at Trader Joe's, and you know that before I became a teetotaler, I used to bring out for guests my very top-shelf alcohol, which was those fancy margaritas in a can from Trader Joe's.
[Regina] (6:17 - 6:25)
It was fancy, and I was skeptical, I have to say, but you convinced me. They're surprisingly yummy, given that they're in a can.
[Kristin] (6:25 - 6:46)
They're actually very good. They're in a can. And to be fair, I would pour it into a fancy glass for you.
[Regina]
Classy. Classy.
[Kristin]
I've now swapped out the margaritas, as you know, for Trader Joe's spinach and kale juice in my new life.
Not quite as much fun, but I still put it in a fancy glass, and I've now added an umbrella, so that makes it even more fun.
[Regina] (6:46 - 6:54)
Yeah, which makes it more festive. Yeah, exactly. I don't get quite the same buzz for the juice, but I do feel more virtuous, so that's a high.
[Kristin] (6:54 - 7:22)
It's almost the same. I feel, yeah. But going back to one of those, the margaritas in a can, it looks like one drink, right?
A single can. That's actually two standard drinks.
[Regina]
No way.
[Kristin]
Yeah. So, if you drink one of those on a Friday night…well, first of all, if you drank one of those on a Friday night, Regina, you'd be keeled over on my living room floor. But, in general, if a person drinks one of those on a Friday night, they would have actually hit the new Canadian drinking limits for the week with just that one drink.
[Regina] (7:23 - 7:24)
With that one can.
[Kristin] (7:24 - 7:25)
Because it's two. Yeah.
[Regina] (7:25 - 7:35)
Interesting. I always pictured Canadians have a lot of heavy drinkers, like beer drinkers, so what is Canada thinking? Do they really expect people to get down to two a week?
[Kristin] (7:35 - 7:51)
I'm guessing this is more aspirational than realistic, at least for some people, but the guidelines do reflect some changing thinking about the risks and benefits of light to moderate drinking, and that's exactly what we're going to talk about today on this podcast.
[Regina] (7:51 - 7:57)
But first, light to moderate drinking, you've not defined that either, so what do we mean by that?
[Kristin] (7:57 - 8:24)
Right, I was afraid you were going to ask me that. There's no standardized definitions of what we mean by that, and this is part of the whole problem with this line of research. It's messy.
People define it very differently in different studies. For the purposes of today, just to give you a ballpark, we're going to say it's roughly an amount between the old Canadian guidelines and the new Canadian guidelines, so women drinking maybe two to 10 drinks per week, and men in that two to 15 drinks per week range.
[Regina] (8:24 - 8:26)
And I can drink all of those in one night, right?
[Kristin] (8:27 - 8:34)
No, that would be considered binge drinking. We’re definitely talking about an amount spread out over the week, not all in one sitting.
[Regina] (8:35 - 8:43)
Moderation, I get it. All right, so you said 10 for women, 15 for men.
[Kristin]
At the top end, yeah.
[Regina]
Right, and so above that, that's heavy drinking.
[Kristin] (8:43 - 9:17)
Again, these boundaries are not standardized, so different people are going to define heavy drinking in different ways, but I would say once you start to get up into that range, you are starting to get into that heavy drinking range, and heavy drinking is unequivocally bad for you. It increases your risks of everything from heart disease to osteoporosis. But in that light to moderate drinking range, the risk-benefit equation is less certain.
Light to moderate drinking definitely has health risks. It increases your risk of certain cancers, at least somewhat. In fact, that was the topic of a recent Surgeon General report.
[Regina] (9:17 - 9:19)
Hmm, so alcohol, not a vitamin.
[Kristin] (9:19 - 9:48)
Not a vitamin, right, yes. I mean, on the cancer, there's definitely debate about which cancers and how much your risk is increased.
[Regina]
Yeah, maybe something for a future episode.
[Kristin]
Definitely, we could tackle that in a future episode. This is actually why I became a teetotaler. For me, when I got the breast cancer diagnosis, it completely shifted my personal risk-benefit equation.
I am just not willing to take any chances with something that's known to increase cancer risk, even if the risk is small.
[Regina] (9:49 - 9:56)
I think that makes complete sense, because we all have our personal risk-benefit equations. Oh, right, right. And that may change over time.
[Kristin] (9:56 - 10:22)
Mine shifted, and I'm a person, you know, my personality, I need to err on the side of caution, especially with this kind of tangible risk right in front of me. I think other people in my position might have made a different decision that would be perfectly reasonable, but I have a low risk tolerance at this point.
[Regina]
Right, right.
[Kristin]
I should also point out, besides cancer, alcohol, even in moderate quantities, has other risks, addiction, accidents, impaired judgment, right?
[Regina] (10:23 - 10:23)
Drunk texting exes. Does that count?
[Kristin] (10:24 - 10:25)
That is an important risk, yes.
[Regina] (10:26 - 10:39)
Well, benefits, you talk about risks and benefits. We haven't talked about the benefits. I'm guessing relaxation, right?
You have to actually get out of your house if you are going to a happy hour.
[Kristin]
Right, right.
[Regina]
And your friends get funnier.
Some of them, they get a lot funnier.
[Kristin] (10:39 - 11:33)
Yes, my friends definitely get funnier, yes. They don't get as funny with the Trader Joe's spinach and kale drink.
[Regina]
No, they don't.
[Kristin]
Yeah, I think the point is, yes, it can be de-stressing, it's a social lubricant, and people take risks to have some fun in life. So, there are definitely benefits socially and for relaxation, yeah. But I think what's really tipped the scales in favor of light to moderate drinking is this long-standing belief that it improves heart health.
If you can offset some heart disease with light to moderate drinking, that might balance out some of these other risks of drinking that we've talked about.
[Regina]
That makes sense.
[Kristin]
And, you know, I might have factored that into my own risk-benefit equation before my diagnosis.
You know, if I was having a nice glass of red wine on a Friday night, it's not that I felt virtuous, but I definitely felt a little less guilty thinking, you know, maybe I'm doing some good for my cardiovascular system.
[Regina] (11:33 - 11:37)
I think rationalization is a strong motivator.
[Kristin] (11:37 - 12:10)
I don't think I'm the only one, right? But there is a lot of history behind this belief that light to moderate drinking is good for the heart.
[Regina]
All right. History. I love it.
[Kristin]
So, this goes back to the mid-20th century.
There were some large, well-done cohort studies where they asked people about health habits like drinking, then they followed them for a long time to see who developed heart disease. And they found that light to moderate drinkers actually had lower rates of heart disease than either the non-drinkers or the heavy drinkers. It actually formed what we call a J-shaped curve.
[Regina] (12:10 - 12:24)
J-shaped curve. Right. For our listeners, maybe you can explain a little bit of what a J-shaped curve is, how it's different from a U-shape, especially because we hear about that U-shape.
[Kristin] (12:24 - 12:48)
Right. So, imagine you plot heart disease risk on the Y-axis and drinking level on the X-axis. You end up with a J-shape because you start a little higher, the non-drinkers have a bit of a higher risk, and then there's a dip, your risk drops for those light to moderate drinkers. This forms the bottom of the J, but then it spikes up for the heavy drinkers, and this forms the back of the J because heavy drinkers have much higher risk than non-drinkers, so it's not a U.
[Regina] (12:49 - 13:13)
And it's that bottom of the J that we're really surprised at, right? So, non-drinkers have a certain risk, we expect that, but then when we go to the light to moderate drinkers, it's actually lower, and we're really surprised by that.
[Kristin]
Yeah, exactly.
[Regina]
So, if we're talking about the difference between not drinking and light to moderate drinking, how do we know that about people's behaviors? Do they just self-report what's behind that?
[Kristin] (13:13 - 13:31)
Yeah, it's usually just self-report, usually a survey. I mean, obviously that's not ideal because not everybody is going to be truthful, right?
[Regina]
Yeah, sensitive.
[Kristin]
Yeah, it's a sensitive question, but how else are we going to get the information? I mean, are we going to go scour people's recycling and trash and count the bottles? I mean, that would be much harder.
[Regina] (13:31 - 13:36)
That would be much harder, a little weird, but more interesting, I think, you know, as a study design.
[Kristin] (13:36 - 13:38)
Right, probably illegal, right?
[Regina] (13:38 - 13:52)
Illegal, yeah, right. I'm not signing a consent for you to come pick through my trash. That non-drinker group is kind of tricky because doesn't this include people who maybe previously drank but stopped drinking because of health problems?
[Kristin] (13:52 - 14:14)
Yes, we have to worry about this. I mean, I'm a perfect case in point.
[Regina]
You are.
[Kristin]
I am in the non-drinker group, but I am there as a direct result of a health problem. Yeah, it's reverse causality.
[Regina]
It is.
[Kristin]
Non-drinking didn't cause the health problem, rather the health problem caused me to move categories and become a non-drinker, but this is going to make the non-drinker group look less healthy, right?
[Regina] (14:14 - 14:16)
And I think you're not alone in this.
[Kristin] (14:16 - 14:16)
No, no, no.
[Regina] (14:16 - 14:22)
There are a lot of people who are going to be in this category and that kind of mucks things up a little bit, doesn't it?
[Kristin] (14:23 - 14:54)
It's a tough problem to overcome analytically. Of course, researchers have tried to do things like let's ask people about former drinking and we'll take out the former drinkers from that group or ask people about diseases and take out the sick people from that group. In general, they still do find the J-shaped curve.
It's really quite a robust finding. It's been replicated over and over again, this J-shaped curve. Across many different studies, many different populations, it's pretty robust.
[Regina] (14:55 - 15:01)
So, any kind of alcohol? Does it matter if I have like a gin and tonic instead of an expensive glass of wine?
[Kristin] (15:01 - 15:16)
It's unclear because a lot of studies don't actually ask people specifically about the kind of alcohol or if they did, you know, it's a messy variable and they've had mixed results. Some have found a stronger effect for wine, but others have found that the type of alcohol doesn't matter.
[Regina] (15:16 - 15:17)
It might just be alcohol. Interesting.
[Kristin] (15:17 - 15:24)
Right. Some people, of course, think it's the antioxidants in wine, especially red wine.
That's good for the heart. That's one of the theories.
[Regina] (15:25 - 15:38)
If it's just antioxidants, can't I just have some kale and blueberries and green juice instead?
[Kristin]
Not as much fun.
[Regina]
No.
But yes. Any other biological explanations, then, if it's not?
[Kristin] (15:38 - 15:41)
All sorts of fun theories, Regina. Some more plausible than others.
[Regina] (15:41 - 15:42)
Okay.
[Kristin] (15:42 - 16:31)
So, we have some short-term experimental studies where you can show if people drink alcohol, their blood vessels relax a little. It's called vasodilation.
[Regina]
They relax?
[Kristin]
Yes.
[Regina]
Oh, that's so cute.
[Kristin]
Right. And also, it has some blood thinning effect, at least in the short term. So there's some plausible theories of what might be going on. Okay.
I also saw a study recently where researchers scanned people's brains using a PET scan. They found that the light to moderate drinkers had reduced activity in the amygdala of their brains compared with non- or minimal drinkers.
[Regina]
Now, amygdala, what is that again?
[Kristin]
That's the fight or flight center of the brain. Ah, right. So they were speculating that maybe if you drink moderately, you will have less of a stress reaction, less of a stress response, that was the idea?
[Regina] (16:31 - 16:40)
You also get beer goggles, right?
So, beer goggles, of course, send you home with the wrong person, let's just say that everyone looks better.
[Kristin]
But you will be less stressed about it. You'll care less.
[Regina] (16:40 - 17:11)
Oh, well, this is perfect then. This is a great combination.
Okay. I'm not a biologist, of course, so maybe I shouldn't laugh, but maybe all these things are completely plausible, but to me, it just sounds like those kids' stories, those just-so stories, right, why the leopard got its spots or why the giraffe's neck is so long. You have this crazy story that just happens to perfectly explain the results, and it justifies what you really want to do, which is have a drink and then say, hey, it's for my health.
Yes. Yeah.
[Kristin] (17:11 - 17:31)
Yeah. Regina, you're totally right. Some of these explanations are hand-wavy for sure, but by the late 20th century, a lot of scientists were really convinced that there is a causal relationship here.
And I want to read you a quote from a 1993 paper in the New England Journal of Medicine, one of the most prestigious journals.
[Regina] (17:32 - 17:38)
Oh, wait, I'm going to interrupt. Ninety-three, this is ancient. Ancient history in here.
This is before we were in grad school together.
[Kristin] (17:38 - 18:27)
Not ancient. Yes, in 1993, we were too young to be in grad school, but not ancient.
[Regina]
We're still too young to be in grad school.
Okay. All right. Go ahead.
[Kristin]
All right. So this paper was by Arthur Klatsky and Gary Friedman, who did a lot of early work in this area using the Kaiser database, and this database includes a large number of people from California who were insured by Kaiser.
And they managed to replicate the J-shaped curves using this data set, and I have a lot of respect for both of those researchers. Gary Friedman, who sadly passed away a few years ago, he had the office across from me at Stanford for many years when he was an emeritus professor. Here's the quote, though, from 1993.
There now seems little doubt that alcohol exerts a protective effect against coronary heart disease.
[Regina] (18:27 - 18:33)
Wow. Exerts a protective effect. That's really strong language, especially for scientists.
[Kristin] (18:33 - 18:34)
Yeah.
[Regina] (18:34 - 18:56)
Very causal language.
[Kristin]
Very causal.
[Regina]
But we're talking about observational studies here.
You mentioned this is a big Kaiser database, so this is for people who chose to drink or not to drink. We can't get causation from that, though. Maybe the people who drink are just different than people who don't drink in other ways that affect their health, besides just the drinking.
[Kristin] (18:57 - 19:16)
Yes, absolutely, Regina. There's good data to show that light to moderate drinkers, on average, they're more educated and they are wealthier than non-drinkers, so lots of differences between those groups.
[Regina]
I think I would have pictured it the other way, you know?
[Kristin]
It's a little counterintuitive, but if you sort of think about it, alcohol costs money, right? It takes leisure time, so it actually does tend to track with wealth.
[Regina] (19:16 - 19:20)
That makes sense. We have time to sit around and have a nice glass of wine.
[Kristin] (19:21 - 19:23)
Or Trader Joe's kale and spinach juice.
[Regina] (19:24 - 20:00)
Hmm. Okay. All right.
But that means that comparing drinkers to non-drinkers is really not a fair comparison here. In stats lingo, we call these things education, income, right, what you're talking about here, we call them confounders. Confounding variables.
We have to throw in a little jargon in here. Let's talk about confounders, if you don't mind, but income and, you know, education are kind of boring, and I might fall asleep, so more fun example, and bringing it back to meat. How about French fries?
[Kristin] (20:00 - 20:01)
Oh, French fries, yeah. We all love French fries, of course.
[Regina] (20:01 - 20:02)
Uh-huh.
[Kristin] (20:02 - 20:02)
Yeah.
[Regina] (20:03 - 20:07)
People who drink in moderation, willpower.
[Kristin] (20:07 - 20:19)
Right. Yeah. If you're a person who can drink in moderation, maybe you can moderate a lot of things in your life, like you can stop at five French fries rather than eating the double-supersized McDonald's bag.
[Regina] (20:19 - 20:26)
I don't understand people like that. I can't stop at five fries. I think we need to patent this, the French fry index.
The French fry index.
[Kristin] (20:26 - 20:30)
The right index for willpower. That could be a variable in everybody's papers, yes.
[Regina] (20:30 - 20:39)
So, in these early studies, how do they deal with these confounders? I'm guessing they used regression methods, statistical adjustment.
[Kristin] (20:41 - 21:06)
The researchers used regression with statistical adjustment, exactly. And when you read papers or you read news stories, you're going to see things like they'll say, they controlled for, or they adjusted for, or they accounted for all of these confounding variables, socioeconomic status, education, French fries. Right.
That language is usually code for, they did statistical adjustment with regression.
[Regina] (21:06 - 21:34)
So I think, Kristin, we need to take a little statistical detour here and talk about statistical adjustment. But first, let's take a quick break.
Kristin, we've talked about your medical statistics program. It's just a fabulous program available on Stanford Online. Maybe you can tell listeners a little bit more about it.
[Kristin] (21:34 - 21:48)
It's a three-course sequence. If you really want that deeper dive into statistics, I teach data analysis in R or SAS, probability, and statistical tests, including regression. You can get a Stanford professional certificate as well as CME credit.
[Regina] (21:49 - 22:28)
You can find a link to this program on our website, normalcurves.com.
Welcome back to Normal Curves. We've been talking about observational studies on alcohol and how investigators statistically control for confounders like education and income and French fries.
Kristin, I think people throw around this term statistical adjustment without always understanding what we mean by it. And I think sometimes the scientists themselves maybe don't even understand.
[Kristin] (22:28 - 22:36)
Oh, yeah, Regina, I think that's absolutely correct. A lot of scientists don't necessarily understand what's happening in this mysterious black box.
[Regina] (22:36 - 22:39)
Black box. That's what it is. I think it's really the key to understanding all this.
[Kristin] (22:40 - 22:40)
Oh, I agree, yeah.
[Regina] (22:40 - 23:01)
Yeah, yeah. It's the tool that investigators have been using to declare causality that alcohol leads to better health rather than just people who drink tend to be healthier. I want us, if it's okay, to talk through together maybe a simple example of statistical adjustment because you and I know a lot about it.
And I'm going to put it on you, Kristin. Start us off. Go ahead.
[Kristin] (23:01 - 23:49)
First of all, Regina, I want to put a little plug in for our statistics courses on Stanford online for those who want to learn even more about regression. We teach this in our joint course, Demystifying Data. And I also teach this in the medical statistics program that I offer if anyone wants an even deeper dive.
But we'll try to peer under the hood a bit here too with a quick statistical detour. All right. Imagine that we have just two groups. I'm trying to keep it simple. A group of non-drinkers and a group of moderate drinkers.
The drinker group has a lower rate of heart disease, but the two groups are different. For example, the moderate drinkers are better able to moderate their French fry intake. They eat an average of just 10 French fries per week, and that non-drinker group, they have 100 on average.
[Regina] (23:49 - 24:19)
Okay. Thank you. I like that.
French fry, I should say, French fry intake, people, is not a variable that we commonly measure. This is better, more fun in this hypothetical example. And we're going to trademark this patent, the French fry intake.
[Kristin]
Oh, absolutely. Yes.
[Regina]
The point is, it's not a fair comparison because we know that people who eat fewer French fries are healthier.
They're less prone to clogging their arteries with the yummy, yummy French fry. Right.
[Kristin] (24:19 - 24:35)
And this is where the regression model comes in. We can use the model to tease out how much of the effect, this is the lower rate of heart disease in the drinkers, how much of that is due to drinking versus how much is just due to French fry abstention.
[Regina] (24:36 - 24:40)
The technical term, of which I am not one. I am not a French fry abstainer.
[Kristin] (24:40 - 24:55)
All right. All right. Let's get a little bit behind the scenes here and talk about the model.
The model takes us sort of mathematically into a hypothetical world in which the drinkers and the non-drinkers have identical French fry intakes.
[Regina] (24:55 - 25:05)
I like this. I like the hypothetical world idea. That would be if you started eating as many French fries as me, but as in your previous life, we still drank different amounts.
[Kristin] (25:05 - 25:23)
Exactly. Of course, I'm not actually eating more French fries and the drinkers and non-drinkers aren't actually consuming identical amounts of fries, but we can use the math to estimate how big of an effect alcohol would have on heart disease if we were in this hypothetical world where everybody ate the same amount of fries.
[Regina] (25:24 - 25:26)
Thanks to math. Yay, math.
[Kristin] (25:27 - 25:39)
Yay, math. And the other great thing about math, because we're using math, we can make our hypothetical world even more elaborate and throw into our model other potential confounders, like the boring ones, income, education.
[Regina] (25:39 - 26:05)
The income, the real ones, I should say. Kristin, when we're describing it this way, it really sounds perfect, doesn't it? It sounds very magical.
We put it all in the computer. The computer does all the work. We're not doing this by hand.
And the way we're describing it makes it feel like we now no longer need to worry about any of these other things, age, whatever, income, diet, and that's really not true.
[Kristin] (26:05 - 26:46)
So I wanted to point that out. That is not true. Statistical adjustment is not perfect, and this really does surprise a lot of people.
I have this very distinct memory of being in a seminar, and I think it was a statistician giving the seminar and a bunch of scientists, yeah, non-statisticians in the audience. And he was talking about how they did a regression model and, oh, but we still have residual confounding by, I don't remember the variable, let's say it was smoking, like, but we haven't, you know, we put smoking in the model, but it's not completely taken care of. And there was like this audible gasp in the audience of like, wait, we put it in the model, it's not fully taken care of?
They, you know, people didn't realize that putting it in the model doesn't mean you've stripped away all the confounding.
[Regina] (26:46 - 26:50)
They lost their statistical adjustment virginity, I think, at that moment.
[Kristin] (26:50 - 27:12)
That's the moment, yes. Right. But statistical adjustment, it is imperfect because our models and our measurements are imperfect.
On the models, we pretend that our variables have simple mathematical relationships, usually straight line, like every one additional French fry increases the risk of heart disease by exactly, you know, 0.05 percent.
[Regina] (27:12 - 27:16)
It would be nice if nature were that perfect and simple, but it's not.
[Kristin] (27:16 - 27:44)
We also measure variables imperfectly. And I think my favorite one is when people say, oh, I adjusted for diet. Because what does that even mean?
[Regina]
Diet. Right. Diet is not a variable.
[Kristin]
There's not one specific variable that captures all of your diet. Like, what did they put in the model? French fry.
French fry intake, right? Fruits and vegetable intake, how many calories? There's all these different potential dimensions to diet.
None of these perfectly captures your diet.
[Regina] (27:45 - 27:57)
Right. Your French fry intake doesn't tell you everything about your diet. Because I put French fries on my kale salad.
[Kristin]
You do not.
[Regina]
I do. It's yummy that way.
And I feel like it balances out.
[Kristin] (27:57 - 27:58)
Oh, wow. Yeah, you're interesting.
[Regina] (27:59 - 27:59)
Thank you.
[Kristin] (28:00 - 28:15)
Right. You know, it's hard to capture all of your diet in one or a few variables. Yep.
And of course, people don't report their diets accurately. Like, I might not want to admit about the chocolate I had for breakfast. So, there's a lot of noise in that diet variable.
[Regina] (28:16 - 28:32)
So, all of these sorts of things, they really make this hypothetical world that you're describing, it really makes it blurry and kind of wonky. Statistical adjustment, I guess, you and I are saying, is not a magic wand, right?
[Kristin] (28:32 - 28:56)
Math is amazing. It's not magic.
[Regina]
It's not magic.
[Kristin]
Otherwise, we would be magicians and not statisticians. That might be more fun.
[Regina]
Presto.
[Kristin]
Because the math is not magic, what we're saying is it's impossible to strip away all of the confounding. We're always going to have some of what I mentioned before, this leftover or residual confounding due to this blurriness.
[Regina] (28:56 - 29:37)
So, not just that, but also things we did not measure, the unmeasured confounding. If we don't measure it, we can't even try to control for it. You can't put it in the model.
And sometimes these are the most important thing. Like we mentioned, willpower, right? So, if you are drinking alcohol in moderation, you probably have a lot of willpower and you're also getting up early to exercise and you're not eating ice cream every day for breakfast.
So, it's the willpower that's really affecting your health and not the drinking. But since we're not measuring willpower, which is really hard to measure, then we can't control for it, right? We're overlooking it.
[Kristin] (29:38 - 30:34)
Exactly. Regina, I like to think of statistical adjustment a little bit like Botox. Bear with me on this one. Okay.
[Regina]
I cannot wait. Okay. Go ahead.
[Kristin]
Botox is an effective tool. It works. Similarly, statistical adjustment, also an effective tool.
It works. But nobody expects Botox to make you completely wrinkle-free. And yet everybody expects statistical adjustment to make you confounder-free, right?
But with Botox, you treat a single wrinkle and it's going to soften that wrinkle, but it's not going to remove it 100%. You're going to get some leftover or residual wrinkling.
[Regina]
I like it.
[Kristin]
And then similarly, you're not going to get, I don't think when they give Botox, they treat every wrinkle in your face. There's going to be some untreated wrinkles, and of course, those will still be there. That's like your unmeasured confounding.
In the same way, statistical adjustment doesn't take away all of the confounding.
[Regina] (30:34 - 31:08)
The unmeasured confounding. All right, Kristin, you win a prize for this. How can I give you like gold medal, bar for this?
I think also you've ruined Botox for me and statistical adjustment because I'll never be able to untangle them, get them straight in my head again. But that's okay because I loved this so much. And while you're doing this, I want to take it one step further, right?
Take your crazy analogy, metaphor. With both of them, it is really easy, really possible to overdo it. Overdo it and you overdo Botox, it is just unnatural, right?
[Kristin] (31:08 - 31:09)
Right.
[Regina] (31:10 - 31:11)
Crime against humanity.
[Kristin] (31:12 - 31:19)
You can also overdo statistical adjustment. Yeah, you can over adjust and actually that's a statistical topic for another day.
[Regina] (31:19 - 31:38)
Okay, all right. So, we have learned about problems with confounding and Botox and statistical adjustment. I want to bring all of this back now to what we were talking about before, which is these giant observational studies that we're using statistical adjustment then to declare causality.
Bring us back to that.
[Kristin] (31:39 - 31:57)
Yes, I think the scientific community was overly confident in their interpretation of those observational studies. In reality, it's statistically quite plausible that the associations that remained after adjustment could have been the result of both residual and unmeasured confounding.
[Regina] (31:57 - 32:00)
Oh, so a bit of wishful thinking maybe.
[Kristin] (32:01 - 32:26)
A lot of wishful thinking. And Regina, I have a little confession to make here.
[Regina]
Oh dear.
[Kristin]
I engaged in some wishful thinking too, I think, when it comes to drinking.
Yes. I mean, everybody wants alcohol to be good for you, right? I mean, it tastes good.
[Regina]
No, it doesn't.
[Kristin]
No, maybe not for you, but for most people, right? It's fun.
So, when I was writing for Allure magazine back in the 2000s, I did write a few stories about the potential health benefits of light to moderate drinking.
[Regina] (32:26 - 32:36)
First of all, I just have to say I love that you wrote for a beauty magazine for so long, but I am guessing this was not you sharing your bartending recipes, right?
[Kristin] (32:36 - 32:38)
Pour the Trader Joe's margarita in a glass? No.
[Regina] (32:38 - 32:42)
Not getting big bucks for that one. No, no, no.
This was a health column.
[Kristin] (32:42 - 33:18)
Yes, this was for my health column, and the column was targeted at young women. So, I wrote about weight gain and not heart disease, more relevant for that group, but of course, weight and heart disease are related. I wrote about two studies that involved thousands of women.
One was in the U.S., and it followed women for 13 years, and one was in Denmark, and that followed women for five years. The U.S. study showed that light to moderate drinkers gained less weight on average than non-drinkers, and the Danish study showed that light to moderate drinkers gained less belly fat, less waist girth than non-drinkers.
[Regina] (33:18 - 33:29)
Okay, less weight gain, less increased belly fat, but I noticed you didn't mention by how much. You're not telling me how much.
[Kristin] (33:29 - 33:42)
Statisticians always have to ask how big was the effect. So, it wasn't huge. I'm talking a few kilograms or a few centimeters around the waist, but not trivial, enough that I thought it was important enough to write about.
[Regina] (33:42 - 33:54)
Mm-hmm. But so, come on, belly fat and alcohol. Is there a biological explanation behind this?
I'm picturing a Bunsen burner, right? So, I drink the alcohol, and then it burns my belly fat.
[Kristin] (33:55 - 34:10)
Right, right, right. This is, you know, possibly wishful thinking, but I do want to give the researchers a lot of credit here. They were pretty cautious in their conclusion, so they weren't coming out and claiming that this was a causal association.
[Regina]
Okay.
[Kristin]
They did suggest or hint at some potential causal explanations, though.
[Regina] (34:10 - 34:11)
Like?
[Kristin] (34:11 - 34:36)
Things like, okay, maybe women, when they drink, they eat less. I don't find that too implausible. Like, I might have chosen a cocktail over an appetizer when I was still drinking.
[Regina]
Or over dinner.
[Kristin]
Yeah, maybe, yeah, probably. And then they had some more biological explanations that, yeah, maybe are a little bit hand wavy.
Like, one of them said maybe it's a thermogenic effect. That's kind of like the Bunsen burners, actually.
[Regina] (34:36 - 34:36)
No way.
[Kristin] (34:36 - 34:42)
That you're burning off calories in the form of heat. You might get a little warm and flushed from the alcohol.
[Regina]
Nope.
[Kristin]
You're not buying it?
[Regina] (34:42 - 34:48)
No, I'm not buying that. It just, it still sounds like confounding. What we were talking about, that willpower that we were talking about before.
[Kristin] (34:48 - 35:16)
It could be just the willpower or some kind of other confounding. Yeah, I mean, they did adjust for all the typical confounders we've talked about, socioeconomic status and so on. But as we've talked about, this adjustment isn't perfect.
No, no. I think the bottom line on all of these studies is that light to moderate drinkers, they just look healthier than non-drinkers in a host of ways. But it's a big question mark as to whether these relationships are causal.
And it's really hard to answer that with an observational study.
[Regina] (35:16 - 35:59)
Observational study, exactly. This is why we need randomized, controlled trial. And we just, we need a giant study, right?
Where we take all the 13-year-olds in the U.S. and we randomize them when they're preteens. And one group, we say, hey guys, you get to drink for the rest of your lives, right? Drink a day.
And the other, we say, oops, sorry. You are forever dry and abstaining, no drinking ever. This is good.
Why? Because we remove all of these confounders because they didn't get to choose. So we're randomizing.
We follow them their entire lives. We check in right before they die, see how they're doing. Boom, done.
[Kristin] (35:59 - 36:00)
We've answered the question.
[Regina] (36:01 - 36:02)
You can thank me later. Okay, yeah.
[Kristin] (36:02 - 36:05)
Well, good luck with the ethics committee on that one, Regina.
[Regina] (36:06 - 36:07)
It's good, though. Has anyone tried it?
[Kristin] (36:07 - 36:26)
Well, no one has tried that study, but there have been a few attempts to do randomized trials. But as you can imagine, these are really hard studies to do because most people don't want to be randomly assigned to drink or not. Most people want to choose for themselves.
And then, of course, there's like the ethical issues with giving people booze in a study.
[Regina] (36:27 - 36:31)
Mm-hmm, right. But you did hint earlier about a couple of studies.
[Kristin] (36:32 - 37:05)
There have been a few attempts, and I want to talk about two of them, one in the U.S. and one in Israel. All right, let's start with the U.S. one first. Okay.
So, there was a randomized trial planned in the U.S. sponsored by the National Institutes of Alcohol Abuse and Alcoholism. This is at the NIH, the National Institutes of Health. And this was going to be a six-year worldwide trial where they randomly assigned people to have either one standard drink per day or no alcohol.
They were going to enroll about 8,000 adults. The outcome is cardiovascular disease or death.
[Regina] (37:06 - 37:18)
8,000? You said nearly 8,000 people worldwide. And what did you say, six years?
[Kristin]
It was planned for six years.
[Regina]
That is a lot of booze.
[Kristin]
A lot of booze.
[Regina]
And that's also really expensive.
[Kristin] (37:18 - 37:28)
Very expensive. It was going to cost about $100 million. Actually, though, it was canceled in 2018 after it had enrolled just a handful of participants.
[Regina] (37:28 - 37:30)
Oh, this is why I have not heard of it.
[Kristin] (37:30 - 38:07)
Yes. Okay, what happened? Okay, some investigative journalists uncovered that because this trial was going to be so expensive, some employees from the NIH, they went out and solicited money for the trial from alcohol companies like Anheuser-Busch.
NIH employees not really supposed to directly solicit money from companies. So, this is part of the reason that the study was shut down. The NIH employees also presented the study to the industry in a very positive way, almost saying, well, we just need to do the study.
And, of course, you should sponsor it because, of course, the results are going to be positive. And we're going to find positive results.
[Regina] (38:07 - 38:09)
And, oh, this might be good for sales.
[Kristin] (38:09 - 38:16)
Yep. And so those companies were going to donate $66 million that created, of course, a conflict of interest.
[Regina] (38:17 - 38:27)
You know, this reminded me a little of when I was writing for the Los Angeles Times, their health section back in the day. I don't know if you know this, Kristin. There's actually an alcohol lobby group.
[Kristin] (38:27 - 38:27)
I did not know that.
[Regina] (38:27 - 39:07)
Yeah, they contacted me and they pitched me. They wanted me to write about the health benefits of alcohol.
[Kristin]
They should have come to me.
[Regina]
They should have. My editors, we talked about it, right? Because we get a lot of pitches in there.
Did we want to do it? But journalists, you know, we like to be independent. We get pitched a lot.
But people who are pitching us, they tend to have vested interest in whatever they're pitching.
[Kristin]
So, did you write it?
[Regina]
Yeah, no, we did not write it.
These same sorts of studies that we're talking about, but they were in this overly positive way and it just felt a little not quite right. So, it reminds me of this thing with NIH.
[Kristin] (39:07 - 39:23)
It's a little similar to this randomized trial. It has a similar feel. It's not that you couldn't have written a very fair and unbiased story and it's not that they couldn't have run a fair and unbiased trial using the alcohol industry's money.
But maybe it feels a little shady.
[Regina] (39:23 - 39:48)
I think shady is a good word for it. I think especially these days we're very sensitive to conflict of interest, right? And it's an interesting question.
It's not unlike what drug companies do all the time. That's true. Right?
They fund studies of their drugs because they're expensive. They're expensive to run. How are you going to get them?
But they set up a firewall, right? So, did they plan anything?
[Kristin] (39:48 - 40:20)
Yes, the companies were going to do the same thing here. They were not going to be involved in either running the study or analyzing the data. And I have to say it's a really interesting question.
I'm kind of torn on this one. I mean, I understand the concerns about conflict of interest. On the other hand, I don't think the mere presence of industry funding necessarily contaminates the study.
There's a way to do it with a firewall and it would have been really valuable if this study had taken place. We might have gotten some answers to this very important public health question.
[Regina] (40:20 - 40:30)
The question we're talking about right now, this is a super bummer. It didn't work out, but you did mention another one. The one in Israel.
Yep. Right? Did they manage to pull that one off?
[Kristin] (40:30 - 40:38)
Some researchers in Israel did pull off a much smaller two-year randomized trial of wine drinking. This was called the CASCADE trial.
[Regina] (40:39 - 40:44)
CASCADE. All right. I'm looking forward to hearing about wine, but in a minute.
First, let's take a quick break.
[Kristin] (40:52 - 41:02)
Regina, I've mentioned before on this podcast, our clinical trials course on Stanford Online is called Clinical Trials, Design, Strategy, and Analysis. I want to give our listeners a little bit more information about that course.
[Regina] (41:03 - 41:12)
It's a self-paced course. We cover some really fun case studies designed for people who need to work with clinical trials, including interpreting, running, and understanding them.
[Kristin] (41:12 - 41:24)
You can get a Stanford professional certificate as well as CME credit. You can find a link to that course on our website, NormalCurves.com, and our listeners get a discount. The discount code is normalcurves10.
[Regina] (41:30 - 41:42)
Welcome back to Normal Curves. We're talking about randomized trials of light to moderate alcohol drinking. Kristin, before the break, you mentioned something called the CASCADE trial.
Tell me more about that.
[Kristin] (41:42 - 42:05)
The CASCADE trial was a two-year randomized trial that enrolled 224 adults with diabetes who were non-drinkers at the start of the trial. They were randomly assigned to drink five ounces of red wine, five ounces of white wine, or five ounces of mineral water, that's the control group, for two years. The results were published in the Annals of Internal Medicine in 2015.
[Regina] (42:06 - 42:12)
You mentioned red wine, white wine, water. This was not blinded, I'm guessing.
[Kristin] (42:13 - 42:18)
No, it would be really hard to blind people because most people can tell if they've had a glass of wine, so they did not try to blind it.
[Regina] (42:18 - 42:23)
Hmm. Still pretty amazing. Like, they pulled off a randomized controlled trial of drinking.
[Kristin] (42:23 - 42:34)
I have to give the authors a lot of credit. This is not an easy trial to do. What'd they find?
The authors reported that wine had some statistically significant benefits.
[Regina] (42:35 - 42:36)
I love the way you say it.
[Kristin] (42:37 - 42:37)
Allegedly.
[Regina] (42:38 - 42:49)
Allegedly. And by statistically significant, you mean, of course, they calculated a p-value, and if that p-value is less than 0.05, they would say, yep, here it is, positive result.
[Kristin] (42:50 - 42:55)
Yes, they use this very traditional criterion of p-value less than 0.05 to define a positive result, exactly.
[Regina] (42:56 - 43:00)
So they found positive results then, right? This is good for my happy hours with my new boyfriends.
[Kristin] (43:02 - 43:17)
It sounds good, but the trial did have some glitches. It elicited quite a few letters to the editor, some critical. I'm not going to go into everything, but I do want to do a deep dive on one of the issues, which is the issue of multiple testing.
[Regina] (43:18 - 43:28)
Multiple testing. Okay, so they did a lot of statistical tests, and this is the whole, if you torture the data long enough, it will confess to anything effect.
[Kristin] (43:29 - 43:33)
Yes, it appears to be a case of torturing the data.
[Regina] (43:33 - 43:40)
Okay, Kristin, you and I talk about this concept of multiple testing a lot, so I say let's illustrate this with an experiment.
[Kristin] (43:41 - 43:42)
An experiment, I love it. On air?
[Regina] (43:42 - 43:58)
On air experiment, live, and let's use my favorite psychic ability, ESP, as an example. So I am thinking of a number between 1 and 20, and Kristin, I want you to use your ESP and tell me my number.
[Kristin] (43:58 - 44:02)
My great psychic powers. Okay, Regina, you are thinking of the number 1.
[Regina] (44:04 - 44:07)
Wrong, but are you just going to like start with 1 and go up in order?
[Kristin] (44:07 - 44:14)
No, I have a strategy. Okay, Regina, you are thinking of the number 20.
[Regina]
You can't just do that backwards.
[Kristin]
I have a strategy. Okay, 5.
[Regina] (44:15 - 44:32)
You are a very logical and methodical person. Still wrong.
3 down.
[Kristin]
All right, 16.
[Regina]
I love it.
You have gone off path, but you're still wrong. Sorry.
[Kristin]
Okay, 11.
[Regina]
Still wrong.
[Kristin]
Okay, 13.
[Regina]
13
[Kristin] (44:32 - 44:33)
I got it? Very nice.
[Regina] (44:34 - 44:58)
13, lucky 13. All right, great. So this experiment, if you and I were reporting the results of this highly scientific experiment for the public, and we were completely transparent, we had this entire sequence so far.
Well, first of all, Kristin, no one is going to mistake you for being a psychic.
[Kristin]
You're saying I shouldn't give up my day job?
[Regina]
Do not give up your day job.
And you got it on how many tries?
[Kristin] (44:59 - 45:00)
It took me six tries.
[Regina] (45:00 - 45:16)
Six tries. Okay, you should not. I'm not sending you my money and asking you who my future husband is or what my life is going to be about.
Okay, and we were completely transparent, and we presented everything. There wouldn't be a problem because we were being transparent.
[Kristin] (45:16 - 45:20)
If we showed everybody all my wrong answers, then they would know that I wasn't actually psychic.
[Regina] (45:21 - 45:27)
Okay, yep, yep. But now imagine that you and I are really clever, and somehow we figured out how to edit this.
[Kristin] (45:27 - 45:30)
Well, that's not trivial to use the editing software.
[Regina] (45:30 - 45:36)
We got a way to go before we're good at that. Yes, we were devious. Let's say we were devious.
[Kristin] (45:36 - 45:37)
Devious, yes.
[Regina] (45:37 - 45:43)
And we cut out all of your wrong answers, and we just jumped right to the right one.
[Kristin] (45:43 - 45:45)
We made it look like I got it on the first try.
[Regina] (45:46 - 45:49)
Yes. Yeah, it'd be a lot more impressive.
[Kristin] (45:49 - 45:50)
That would be a lot more impressive.
[Regina] (45:51 - 45:59)
You could put yourself out there as a psychic, make some money that way. But that would be wrong, Kristin. That would be bad.
That would be the problem.
[Kristin] (45:59 - 46:19)
Right, of course. It's that lack of transparency that would get us into trouble. And bringing this back to CASCADE, something similar may have happened there.
I'm going to put more details in the show notes for those who are curious.
[Regina]
Good.
[Kristin]
And we also discuss this in great detail, actually, this particular trial in our clinical trials course on Stanford Online.
[Regina] (46:19 - 46:22)
Which, if I do say myself, is a terrific course.
[Kristin] (46:22 - 46:42)
If you want to know soup to nuts on clinical trials, if you want that really deep dive, I'm going to send people there. Yeah. But in CASCADE, the authors report five statistically significant results in their paper.
Things like red wine significantly improved HDL cholesterol at two years and white wine improved insulin sensitivity.
[Regina] (46:42 - 46:46)
That sounds promising. Cholesterol, insulin, right?
[Kristin] (46:46 - 47:33)
It sounds impressive, except that it appears that they ran a lot of statistical tests in the background. They report 15 different outcomes in the paper. That's just the ones we know about.
They might have looked at more variables that didn't make it into the paper, but 15 in the paper. And then for each of these variables, they had to do two comparisons because they compared red wine to water, but then they also compared, for each variable, white wine to water. And they checked for differences at different time points.
And if you run a lot of tests, you're going to get lucky sometimes. If wine has no benefits at all, but we compare the two wine groups to the water group on enough different variables at enough different time points, we're going to find a few statistically significant results just by chance.
[Regina] (47:33 - 47:56)
Just by chance. Chance fluctuations, false positives. It's really not that impressive, is it?
Exactly. This is why in clinical trials, you're supposed to designate one or two primary outcomes ahead of time. So you can't just after the fact go through, having to rifle through all your data, cherry pick a few things.
So did they do this? Did they pre-specify a primary outcome?
[Kristin] (47:56 - 48:05)
Okay, so they did pre-specify a single primary outcome in their protocol, but this appears to be a case of what we call outcome switching.
[Regina] (48:05 - 48:08)
Oh, that's a, guys, that's a big no-no. I'm so disappointed.
[Kristin] (48:08 - 48:30)
Yeah, you can go to their publicly available protocol. They did publish it.
They set a single primary outcome ahead of time, but they didn't stick to it. They switched. And then they get these five statistically significant results and they label these in the paper as their, quote, primary outcomes.
But those five outcomes were not pre-specified in their protocol. They switched them.
[Regina] (48:30 - 48:31)
Yes.
[Kristin] (48:31 - 48:31)
They switched them.
[Regina] (48:31 - 48:42)
And so you're, of course, worried that they found these significant results after the fact and then labeled them as primary. Kind of like editing out those wrong answers for ESPs.
[Kristin] (48:42 - 49:06)
Exactly, Regina. Yeah, that's what appears to have happened. And of course, I don't know all the, you know, I don't know what actually happened behind the scenes, but I am not the only person to point out this outcome switching.
So I think there's pretty good evidence of it. And, Regina, I'm looking at the results and to me, when I see these results, now knowing the context, to me, this study now doesn't provide evidence of benefits for wine. I just don't see it there.
[Regina] (49:06 - 49:09)
No, it's not very impressive. Oh, that's so sad.
[Kristin] (49:09 - 49:12)
I know, sorry for all those drinkers out there.
[Regina] (49:12 - 49:26)
Let's take stock. Where are we? We learned one statistical adjustment, not magic.
Observational studies have problems and now we're seeing the limitations of randomized control trials.
[Kristin] (49:26 - 49:28)
It's hard to do them.
[Regina] (49:29 - 49:32)
So do we just give up at this point?
Are we done?
Going home?
[Kristin] (49:32 - 49:52)
Well, there is an interesting and really powerful study design that's being used more and more over the last decade to try to answer questions like this. And I think it's worth spending a little time talking about it.
It's called Mendelian randomization. So you're going to pull that out at cocktail parties so you can sound really smart.
[Regina] (49:54 - 49:57)
Actually, I put Mendelian randomization on my online dating profile.
[Kristin] (49:58 - 49:59)
You did not! What?
[Regina] (50:00 - 50:13)
You gave me this paper to read, right, in preparation for this episode, and I got super into this. And the question prompt on the dating site was, what are you really into right now? And I said I was super into Mendelian randomization.
[Kristin] (50:14 - 50:20)
So what's the strategy there? You're looking for the really nerdy types? I'm not sure that's a great strategy.
[Regina] (50:20 - 50:29)
I am. I like the smart guys. I actually had a few guys message me and say, oh, I love it because I had to go look up what this Mendelian randomization is.
[Kristin] (50:29 - 50:32)
Oh, wow. Then we educated some people. All right, I'm glad.
[Regina] (50:32 - 50:50)
Good. But let's get back to you and why you and I are so into Mendelian randomization right now. For our audience, of course, Mendel is in this term, Mendelian randomization.
And Mendel is the father of genetics. So I'm guessing DNA is in here.
[Kristin] (50:51 - 50:52)
Yeah, genetics is in there.
[Regina] (50:52 - 50:52)
Exactly.
[Kristin] (50:53 - 51:45)
The idea is to view genetic variation as a natural experiment. You don't get to choose what genes you're born with. So you're, in some sense, randomly assigned to them at birth.
And it turns out that you can inherit genes that actually affect your drinking behavior.
[Regina]
Which is crazy, really.
[Kristin]
Yeah, it's kind of wild, but think about it.
One of these is the gene that codes for an enzyme called alcohol dehydrogenase. And it breaks down alcohol.
[Regina]
Oh, interesting.
[Kristin]
So if you have a certain variant of this gene, it affects how you break down alcohol. You don't break down alcohol as well. And so you end up flushed in the face.
You don't feel well. You have all these unpleasant side effects. So people who carry this particular mutation in that gene, they don't tend to drink as much, at least on average, as people who don't carry the mutation because it just doesn't make them feel well.
[Regina] (51:46 - 51:49)
I might have something related to this because when I drink, I get sick really fast.
[Kristin] (51:49 - 51:50)
You don't feel great.
[Regina] (51:50 - 52:10)
All right, so I'm going to go big picture with this. Essentially, when I told you about my great idea of the 13-year-olds that were randomizing, apparently it's already happened. We've all been randomized at birth, but we just don't know it.
Randomized to like be a drinker or not.
[Kristin] (52:10 - 52:20)
Yeah, to some extent. I mean, it's not that we're randomized to drink or not. It's that we're randomized at birth to be more or less prone to drinking, I think would be an accurate way to put it.
[Regina] (52:20 - 52:41)
So this is a natural experiment, right? It's like nature randomized us. We just don't know it.
But I find this a little fascinating and a little scary, too, in here. We have this natural randomization, but explain, Kristin, how this gives us any better evidence than those big observational studies that we're talking about.
[Kristin] (52:41 - 52:50)
Right, right. The idea is that the presence of the gene is not related to any other confounders. That's the key here.
[Regina] (52:50 - 53:02)
So just like a normal randomized trial we were talking about, the confounders are going to be balanced between the two groups. That's what you're saying, right?
Income, education, willpower. Consumption.
[Kristin] (53:02 - 53:03)
Right, right.
[Regina] (53:04 - 53:13)
Being assigned to drink more or less, that's just random, and we don't get to choose it. So those confounders are balanced. Well, this is super powerful.
[Kristin] (53:13 - 53:37)
This is super powerful because now we can compare groups that, in theory, are balanced on all the confounders, just like in a real randomized trial. This allows us to draw causal conclusions. If the group with the mutation has a higher rate of heart disease than the group without the mutation, then we can conclude that having the gene increases the risk of heart disease.
[Regina] (53:37 - 53:44)
Okay. I'm with you on that, but how do we get to actual drinking and heart disease from the genes?
[Kristin] (53:44 - 54:04)
Right. It might help to do a little thought experiment here. Let's imagine for the moment that our gene group completely determines our drinking behavior.
People who have the mutation, let's give them a name. They have the mutation, so they're not drinking? They're the ones who get queasy when they drink.
The queasies.
[Regina] (54:04 - 54:05)
I like it. The queasies.
[Kristin] (54:05 - 54:10)
Let's say they don't ever drink. Okay. And the people who don't have the mutation, what should we call them?
[Regina] (54:10 - 54:11)
Born to drink.
[Kristin] (54:12 - 54:15)
Born drinkers. I like it. Natural born drinkers, like the movie.
[Regina] (54:16 - 54:21)
I love it. Natural born drinkers and the queasies. Right, okay.
It's like a band.
[Kristin] (54:22 - 54:30)
So imagine that the queasies, they follow their genetic destiny and they never drink. Okay. And the natural born drinkers, they all drink.
[Regina] (54:31 - 54:35)
Like a perfect randomized trial. Then you're randomized at birth. Exactly.
[Kristin] (54:36 - 54:54)
And if that were the case, it's easy, right? Because then the gene group and the drinking group are one in the same. Let's just put some made up numbers to it.
Let's say the natural born drinkers have 20% less heart disease than the queasies. This would mean that drinking reduces heart disease by 20%.
[Regina] (54:54 - 55:17)
It makes sense, but real life isn't that tidy, right? Because genetics is not destiny. I might decide I'm going to drink even if drinking makes me really sick because I want to be a party girl.
So not a perfect randomized trial. In reality, it's like we're running, what, an imperfect randomized trial where people don't always adhere to the group they were put into.
[Kristin] (55:18 - 55:32)
That's it. Exactly. So we use the gene groups because it allows us to sidestep confounding, but we can't stop there because we know that compliance is imperfect. Some queasies drink and some natural born drinkers abstain.
[Regina] (55:33 - 55:40)
That dilutes any effect we can see, right? Like you're mixing a whiskey with water. You don't get as drunk.
[Kristin] (55:40 - 55:42)
I like that analogy, Regina.
[Regina] (55:42 - 55:42)
Yes, exactly.
[Kristin] (55:43 - 56:00)
But we can account for this dilution by making an adjustment. All right. For example, let's say that unlike in our thought experiment where the genes explained 100 percent of our drinking behavior, let's say that the gene group explained, say, just 50 percent or half of our actual drinking behavior.
[Regina] (56:01 - 56:05)
And this is something we can figure out from the data, right? We can calculate.
[Kristin] (56:05 - 56:21)
Yes, we have data on actual drinking behavior, and we can figure out how much more alcohol the natural born drinkers consume compared with the queasies, and that allows us to calculate that figure. And let's say it is half. The gene group explains half of the drinking behavior.
[Regina] (56:22 - 56:23)
OK, what do we do with that?
[Kristin] (56:23 - 56:40)
Right. We can use this information to make an adjustment and just, again, put some numbers to it. Let's say, again, that the natural born drinkers have 20 percent less heart disease than the queasies.
We would then estimate that drinking reduces heart disease by 40 percent.
[Regina] (56:41 - 56:50)
It's like we know that our drink is half water and half whiskey, so then we can guess that without the water, we would have gotten twice as drunk.
[Kristin] (56:50 - 56:51)
Yes, I like that analogy.
[Regina] (56:51 - 56:51)
That's perfect.
[Kristin] (56:52 - 57:50)
Yes, that's the idea. I should mention, though, Regina, I just want to give the assumptions, the caveats, the fine print. Important.
Right? This is not as good as a randomized trial. OK, we have made a lot of assumptions behind the scenes in a Mendelian randomization.
We are assuming things like that the gene assignment is truly random, but genes don't always sort randomly if you know a little bit about genetics. We're also assuming things like that alcohol gene doesn't influence other behaviors besides drinking, that it's very specific to drinking. And these things might not always be true.
Right, exactly. I think in this particular case of alcohol and heart disease, the assumptions behind the Mendelian randomization for this case are actually pretty reasonable. I have to say, though, I'm not a fan of all Mendelian randomization studies.
There are some badly done ones out there that where these assumptions are much weaker. We just have a particular case where the influence of genes on drinking is actually pretty strong.
[Regina] (57:50 - 58:10)
Kristin, I love the way that we have traversed this journey here, what we've seen in this episode, because first we saw the limitation to problems with observational studies. And then we talked about the problems with confounding and statistical adjustments and Botox, right? And then we talked about the problems doing randomized trials.
[Kristin] (58:10 - 58:12)
Randomized trials of drinking are really hard.
[Regina] (58:13 - 58:27)
We don't have those. So this, I think, really helps us appreciate why researchers might be so excited about this Mendelian randomization study design, because it answers this need that we've been having.
[Kristin] (58:27 - 58:48)
It's a very powerful idea. Of course, as with any study design, it has to be well executed. You have to meet those assumptions.
But in theory, this is a very powerful design. If you want to get really nerdy about this, Regina, this is also called instrumental variable analysis. And Mendelian randomization is just a special case of that study design using the genes as the instrument.
[Regina] (58:49 - 58:53)
This is true, but I have to say Mendelian randomization sounds sexier.
[Kristin] (58:53 - 59:01)
Yeah. Regina, do not put instrumental variable analysis on your online dating profile. That will not be attracting the right kind of guy.
[Regina] (59:01 - 59:04)
Not at all. OK. So are we going to talk about one particular study or what?
[Kristin] (59:04 - 59:28)
Yeah. There's actually been a bunch of these studies now looking at this question of light to moderate drinking and heart disease. But I want to focus on one that came out in JAMA Network Open in 2022 because I think it's a particularly well-done study.
And we're going to spend a lot of time in this podcast criticizing studies. So I've spent a lot of my career criticizing studies. So I think it behooves us to point out when there's actually a well-done study and to call it out.
[Regina] (59:29 - 59:34)
You're very good at criticizing studies. So if you're saying this is good, it must be really good. There's a lot of things I like about it.
[Kristin] (59:34 - 59:34)
Yeah.
[Regina] (59:35 - 59:35)
This is exciting.
[Kristin] (59:36 - 59:54)
All right. So this study used the UK Biobank, which is this huge data set from the United Kingdom, contains all sorts of variables. It has behavioral variables like drinking, but it also has genetic data and it also has outcomes like heart disease and death.
And they looked at over 370,000 people.
[Regina] (59:55 - 1:00:02)
Yikes. That is huge. 370,000 before the NIH one had planned 8,000, right?
[Kristin] (1:00:02 - 1:00:03)
And that would have been big. Much bigger than that.
[Regina] (1:00:04 - 1:00:04)
This is massive.
[Kristin] (1:00:05 - 1:00:05)
Yeah.
[Regina] (1:00:05 - 1:00:10)
You said UK Biobank, right? So I'm guessing mostly people of European descent.
[Kristin] (1:00:11 - 1:00:19)
Yeah, it is a diverse sample. But of course, because it's in the UK, it's much more representative of people of European descent. So that's a limitation of the study.
[Regina] (1:00:19 - 1:00:20)
All right.
[Kristin] (1:00:20 - 1:00:37)
One thing I really liked about this study is before they got into the Mendelian randomization, they actually took the data they had. They had data about people's drinking behavior and their heart disease outcomes, and they replicated those old analyses that we talked about from the last century, those J-shaped curves. They were able to replicate them.
[Regina] (1:00:38 - 1:00:40)
So this was ignoring genetics, the old-fashioned way.
[Kristin] (1:00:40 - 1:00:54)
It's just going in. And I like this because it shows there was nothing inherently wrong with those old studies. They're able to show that, yes, that J-shaped curve pops out.
Those old studies were using good statistical methods for the time, but those methods just have limitations, right?
[Regina] (1:00:54 - 1:01:01)
And now we've got Mendelian randomization. So what did they find once they added in the genes, the Mendelian randomization part?
[Kristin] (1:01:01 - 1:01:26)
This is the kicker, Regina. First of all, I should say that they used a set of genetic markers as their genetic instrument. So it wasn't a single gene.
They looked at a number of genes that influenced drinking behavior.
[Regina]
Oh, even better.
[Kristin]
It's statistically more powerful to have a whole set of genes.
All right. Here's what they found. They found that people who were genetically predisposed to drink more alcohol had a higher not lower rate of heart disease.
[Regina] (1:01:26 - 1:01:35)
You're kidding. Higher. Yes.
So this goes against everything we've been hearing. The opposite.
[Kristin]
Yes. The opposite.
[Regina]
This is insane.
[Kristin] (1:01:35 - 1:01:52)
No level of drinking was protective. It formed an exponential rather than a J-shaped curve with all the cardiovascular disease outcomes. And this is not just heart attacks, but also strokes, high blood pressure.
The risk always goes up as you drink. It never goes down for all of those outcomes.
[Regina] (1:01:52 - 1:01:58)
But not a line. You said they found an exponential curve. Right.
Right. So explain that exponential part.
[Kristin] (1:01:59 - 1:02:02)
Right. Well, I feel like everybody knows exponential curve now from the pandemic. Yeah.
[Regina] (1:02:02 - 1:02:03)
Okay. Do it anyway.
[Kristin] (1:02:03 - 1:02:26)
Okay. But here's the idea. The risk increases very slowly at first as you drink more.
So as you're going from zero drinks per week, you know, up to like seven drinks per week, that risk increase is pretty small. And then it starts to really escalate. Once you hit about two plus drinks per day, the range right around what we were talking about, heavy drinking, you see this huge exponential rise.
There's a huge increase in risk.
[Regina] (1:02:26 - 1:02:27)
Oh, like there's some tipping point.
[Kristin] (1:02:28 - 1:02:29)
Yeah, exactly.
[Regina] (1:02:29 - 1:02:39)
Yeah, yeah. But the key point is that the risk always increased, never decreased. And this just flies in the face of everything we've been hearing for what like a century.
[Kristin] (1:02:40 - 1:02:40)
Right.
[Regina] (1:02:40 - 1:02:42)
You mentioned there were a lot of other studies.
[Kristin] (1:02:42 - 1:03:08)
Yeah. So there's been other Mendelian randomization studies that have looked at this question, a whole host of them really, and they have all found pretty much the same thing. We are not finding a protective effect for alcohol, even in those low to moderate drinking ranges.
Now, I have to give the caveat. These are not as good as real randomized trials, right? We are relying on a host of assumptions.
But in the absence of randomized trials, I find this evidence pretty convincing.
[Regina] (1:03:09 - 1:03:39)
Wow, this should blow my mind. Kristin, I think we can wrap it all up right now. What do you think?
We're going to evaluate the strength of evidence behind the claim that light to moderate drinking is good for your heart. And how do we do this? We use our smooch rating scale.
It's so much fun. It's like Amazon stars or Uber stars, except they're kisses. One smooch means little to no evidence for the claim.
Five smooches means lots of strong evidence for this claim. All right. Kristin, what do you think?
Kiss it or dish it?
[Kristin] (1:03:40 - 1:03:42)
Am I allowed a half a smooch?
[Regina] (1:03:42 - 1:03:43)
I'll give you half a smooch.
[Kristin] (1:03:43 - 1:04:08)
Okay, I'm going to go one and a half smooches for this question. I am pretty convinced that, in fact, alcohol does not help your heart. But that half smooch in there is the optimist in me.
Oh, yes. Just holding out hope, even though I don't even drink anymore. But I'm still holding out hope that maybe had they done that 8,000-person randomized trial, there'd be something good about red wine for your heart.
Something in there, right? So one and a half smooches. How about you, Regina?
[Regina] (1:04:09 - 1:04:12)
I'm going to go with one smooch, actually.
[Kristin] (1:04:12 - 1:04:12)
Fair enough.
[Regina] (1:04:13 - 1:04:39)
I didn't see anything that was really exciting. I love the Mendelian randomization study. So one smooch.
One smooch for me. And I feel like I'm not losing anything in my life by not drinking. So we also, in this podcast, give methodological morals.
What did we learn today? So it's a little like Aesop's Fables, except nerdier. So do you have a methodological moral from today's story?
[Kristin] (1:04:39 - 1:04:44)
Mine is going to be: Statistical adjustment cannot erase all confounding.
[Regina] (1:04:44 - 1:04:47)
Oh, I love that one. Like wrinkles.
[Kristin] (1:04:48 - 1:04:54)
Right, exactly. Cannot erase. Botox cannot erase all your wrinkles.
Statistical adjustment cannot take away all of your confounding.
[Regina] (1:04:54 - 1:04:55)
And don't overdo it.
[Kristin] (1:04:56 - 1:04:58)
And don't overdo it, yes. How about you, Regina? What's your moral?
[Regina] (1:04:59 - 1:05:03)
When you can't experiment on people, let nature experiment on people.
[Kristin] (1:05:03 - 1:05:06)
I love it. Yes. Mendelian randomization.
[Regina] (1:05:06 - 1:05:18)
I'm just kind of hooked into it. And not just because I put it on my dating profile, by the way. All right.
All right. So Kristin, this has been a lot of fun. I would say cheers.
Let's say cheers. Cheers. With green juice.
[Kristin] (1:05:18 - 1:05:21)
Yes, absolutely. Cheers, Regina.